AI And Wildlife Conservation: Progress And Perils
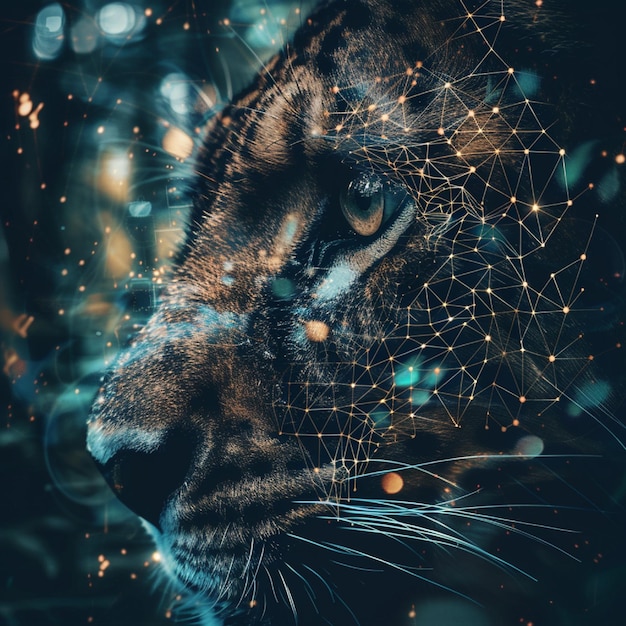
Table of Contents
AI-Powered Monitoring and Surveillance
AI is revolutionizing how we monitor and protect wildlife. Advanced technologies are providing unprecedented insights into animal behavior and enabling more effective conservation strategies.
Real-time Tracking and Poaching Prevention
AI-powered drones, equipped with thermal cameras and sophisticated sensors, are transforming anti-poaching efforts. These drones can patrol vast areas, detecting poachers in real-time and relaying information to ground patrols. Similarly, strategically placed camera traps, coupled with AI-powered image recognition, automatically identify endangered species and flag suspicious activity.
- Successful Deployments: Several African national parks are using AI-powered drone surveillance to significantly reduce poaching incidents. Similar successes are being reported in Southeast Asia, where camera traps and AI are used to monitor endangered tigers and rhinos.
- Limitations: High initial investment costs, challenges with reliable connectivity in remote areas, and potential for technological malfunctions are all limiting factors.
- Integration: Successful implementation requires integration with existing conservation efforts, including community engagement and improved law enforcement. AI is a tool, not a standalone solution. Keywords: wildlife monitoring, poaching detection, AI-powered drones, sensor networks, real-time tracking.
Analyzing Large Datasets for Population Insights
AI algorithms excel at analyzing vast quantities of data from various sources, providing crucial insights for conservation planning. Camera trap images, acoustic recordings of animal vocalizations, and satellite imagery can be processed using machine learning to estimate population sizes, track animal movements, and identify environmental threats.
- Machine Learning Applications: Convolutional Neural Networks (CNNs) are widely used for image recognition in camera trap data, identifying individual animals and estimating their abundance.
- Acoustic Monitoring: AI algorithms analyze acoustic data to identify different species, track their movements, and detect potential threats.
- Data Processing Challenges: The sheer volume of data generated can pose a significant challenge, requiring powerful computing resources and efficient data management strategies. Keywords: population estimation, wildlife tracking, machine learning, image recognition, acoustic monitoring, big data analysis.
AI-Driven Predictive Modeling and Conservation Strategies
Beyond monitoring, AI enables predictive modeling to anticipate future challenges and optimize conservation efforts.
Predicting Habitat Loss and Fragmentation
AI models can analyze environmental data, including climate change projections, deforestation patterns, and land-use changes, to predict future habitat loss and fragmentation. This allows conservationists to proactively identify critical areas for protection and develop targeted interventions.
- Examples: AI models are successfully predicting the impact of climate change on the distribution of various species, helping to identify areas that require urgent conservation action.
- GIS Integration: AI models are often integrated with Geographic Information Systems (GIS) to create detailed maps of predicted habitat changes, facilitating effective conservation planning.
- Limitations: Predictive models are only as good as the data they are trained on. Inaccurate or incomplete data can lead to unreliable predictions. Keywords: habitat prediction, deforestation monitoring, climate change impact, GIS integration, predictive modeling.
Optimizing Anti-Poaching Patrols and Resource Allocation
AI algorithms can optimize anti-poaching patrol routes, maximizing coverage and minimizing resource expenditure. This involves analyzing various factors, such as poaching hotspots, terrain accessibility, and patrol team capacity.
- AI-driven Route Optimization: Algorithms can dynamically adjust patrol routes based on real-time information, such as poaching alerts and environmental conditions.
- Resource Allocation Strategies: AI can assist in allocating resources effectively, ensuring that limited funding and personnel are used where they are most needed.
- Improving Law Enforcement Efficiency: AI-driven tools can significantly enhance the efficiency of anti-poaching efforts, leading to better outcomes with fewer resources. Keywords: anti-poaching strategies, resource optimization, patrol route planning, AI algorithms.
Ethical Considerations and Potential Pitfalls of AI in Wildlife Conservation
While AI offers immense potential, its application in wildlife conservation raises important ethical considerations.
Data Privacy and Security Concerns
The collection and use of large amounts of wildlife data raise concerns about data privacy and security. Protecting sensitive information about animal locations and behaviors is crucial to prevent exploitation and ensure the safety of both animals and researchers.
Bias in Algorithms and Data Representation
Biases in training data can lead to inaccurate or unfair outcomes. For instance, if a model is trained primarily on data from one region or species, it may not generalize well to other contexts.
Over-reliance on Technology and Neglect of Traditional Methods
It's essential to integrate AI with traditional conservation practices, rather than relying solely on technology. Local knowledge and community engagement remain crucial for effective conservation.
Accessibility and Cost of AI Technologies
The high cost and technical expertise required to develop and deploy AI systems can limit access for conservation organizations in developing countries, exacerbating existing inequalities. Keywords: ethical considerations, data privacy, algorithmic bias, data representation, traditional conservation methods, technology accessibility.
Conclusion
AI and wildlife conservation represent a powerful partnership, offering innovative tools for monitoring, predicting, and mitigating threats to biodiversity. From real-time poaching detection using AI-powered drones to predictive modeling of habitat loss, AI is transforming conservation efforts. However, responsible implementation is paramount. Addressing ethical concerns, ensuring data security, and mitigating algorithmic bias are crucial for harnessing the full potential of AI while avoiding unintended negative consequences. The future of wildlife conservation hinges on responsible innovation. Learn more about the exciting potential and crucial ethical considerations of AI and wildlife conservation and join the movement to protect our planet’s precious biodiversity.
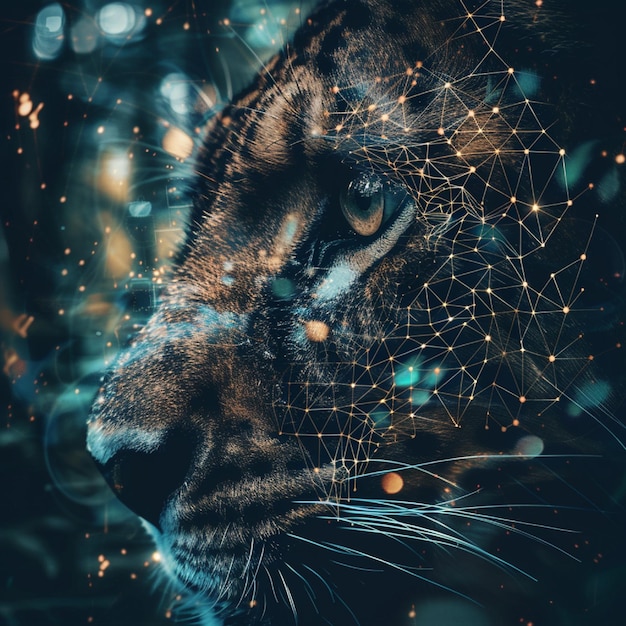
Featured Posts
-
Umpires Call Stirs Controversy In Tigers Game Hinch Seeks Replay
Apr 23, 2025 -
Solutions 30 Analyse Boursiere Et Previsions De Cours
Apr 23, 2025 -
Historic Home Run Performance Yankees Smash Record With 9 Home Runs Judge Leads The Way
Apr 23, 2025 -
Tigers Skubal Throws 7 Shutout Innings Against Brewers
Apr 23, 2025 -
Milwaukees Nine Stolen Bases A New Record Set
Apr 23, 2025
Latest Posts
-
Elizabeth City Road Fatal Accident Leaves Two Dead
May 10, 2025 -
Two Pedestrians Killed In Elizabeth City Road Accident
May 10, 2025 -
Elizabeth Line Gaps In Accessibility For Wheelchair Users And How To Bridge Them
May 10, 2025 -
A Tour Of The Updated Queen Elizabeth 2 2 000 Guests And New Amenities
May 10, 2025 -
Tf Ls Elizabeth Line Accessibility Challenges And Solutions For Wheelchair Users
May 10, 2025