Building A Robust Poll Data System: Challenges And Solutions
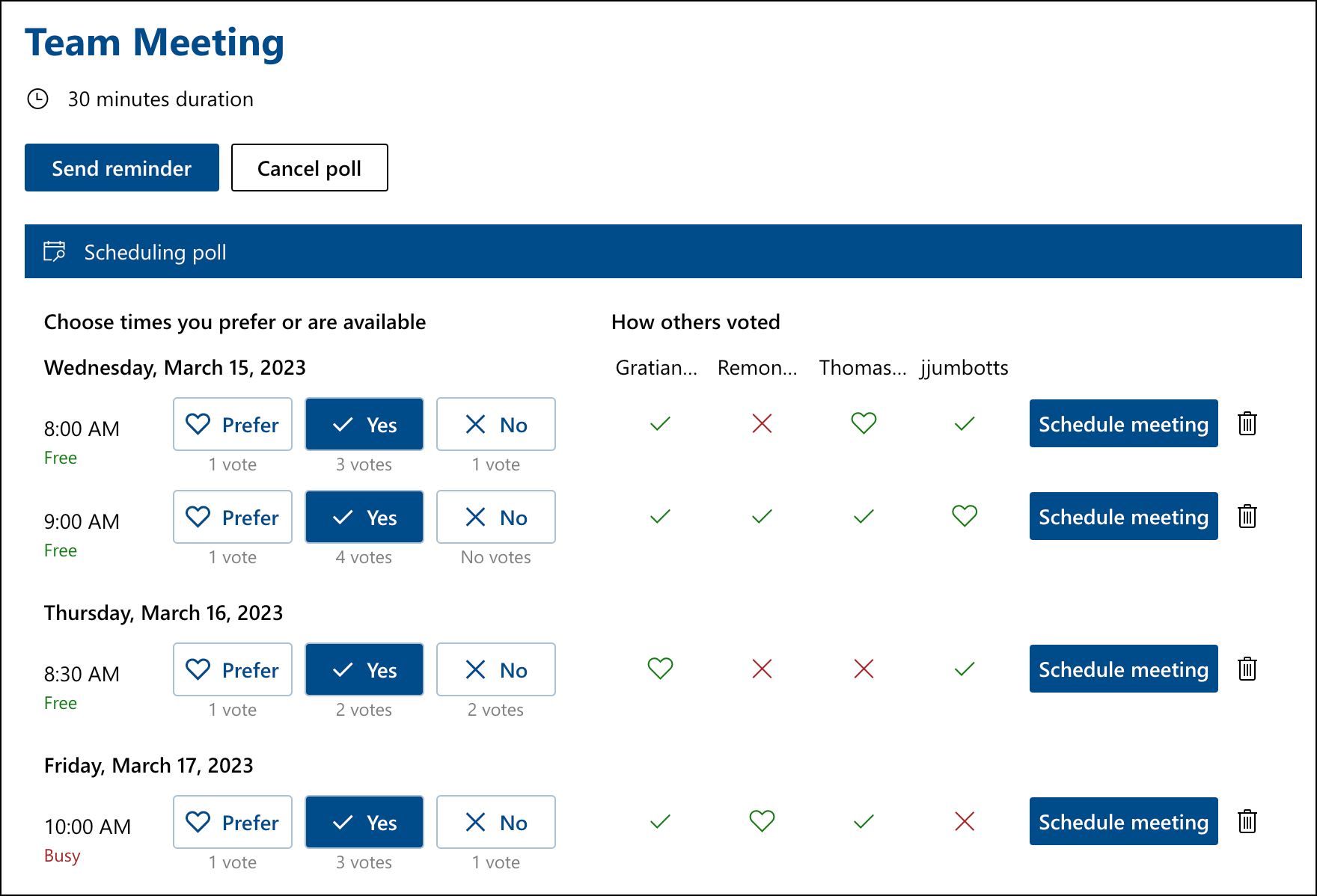
Table of Contents
Data Acquisition Challenges & Solutions
Building a robust poll data system begins with effective data acquisition. This phase presents significant challenges that, if not addressed properly, can compromise the entire process.
Ensuring Accurate Data Collection
Accurate data collection is the cornerstone of any reliable poll. Several factors can introduce bias and skew results, leading to inaccurate conclusions.
- Sampling Bias: A non-representative sample can dramatically distort results. For example, surveying only people with landlines excludes a significant portion of the population, particularly younger demographics.
- Question Wording Bias: The phrasing of questions can subtly influence responses. Leading questions or ambiguous wording can manipulate results.
- Response Bias: Respondents may provide inaccurate answers due to social desirability bias (answering in a way they perceive as socially acceptable), or simply due to misunderstanding the question.
To mitigate these biases, several strategies are essential:
-
Pre-testing questionnaires: Testing the questionnaire on a small group before widespread deployment allows for identification and correction of ambiguous or leading questions.
-
Rigorous sampling methodologies: Employing probability sampling techniques, such as simple random sampling or stratified random sampling, ensures a representative sample of the target population. Using weighted sampling can further adjust for population imbalances.
-
Multiple data collection methods: Combining online surveys with phone or in-person interviews can help reduce biases associated with a single method.
-
Utilize weighted sampling to adjust for population imbalances. This ensures that subgroups are appropriately represented in the final results.
-
Implement quality control checks during data entry. This includes double data entry and validation checks to minimize errors.
-
Employ data validation techniques to identify and correct errors. This may involve using automated checks or manual review of data.
Managing Large Datasets Efficiently
Modern polls often generate massive datasets, posing significant challenges for storage, organization, and processing.
- Data volume: The sheer volume of data from various sources can overwhelm traditional data management systems.
- Data variety: Data might come in various formats (structured, semi-structured, unstructured), further complicating management.
- Data velocity: The speed at which data is collected can be substantial, demanding real-time processing capabilities.
Effective solutions include:
-
Cloud-based databases: Cloud platforms offer scalable and cost-effective storage solutions for large datasets.
-
Data warehousing: Data warehouses provide a centralized repository for storing and managing large volumes of data from multiple sources, facilitating efficient analysis.
-
Data cleaning and pre-processing techniques: This involves handling missing values, correcting inconsistencies, and transforming data into a usable format. Employing database management systems (DBMS) is crucial for this.
-
Implement data encryption and security measures. Protecting sensitive data is paramount.
-
Use automated data import and cleaning tools. This streamlines the data processing workflow.
-
Employ data visualization techniques for efficient data exploration. This helps to identify patterns and anomalies in the data.
Data Analysis and Interpretation Challenges & Solutions
Analyzing poll data accurately and interpreting the results correctly are equally crucial aspects of building a robust poll data system.
Ensuring Data Integrity and Accuracy
Maintaining data integrity is essential to avoid misinterpretations and flawed conclusions.
- Data manipulation: Intentional or unintentional manipulation of data can lead to biased results.
- Data errors: Errors during data collection, entry, or processing can significantly impact the accuracy of the analysis.
Robust solutions include:
-
Regular data audits: Periodic reviews of the data and the analysis process help to identify potential errors or inconsistencies.
-
Implementing version control for data: Tracking changes to the dataset ensures data integrity and allows for recovery from errors.
-
Using robust statistical software: Statistical software packages provide tools for data analysis, error detection, and reporting.
-
Employ cross-validation techniques to verify results. This involves using multiple methods to analyze the data and comparing the results.
-
Utilize statistical significance tests to assess the reliability of findings. This ensures that the results are not due to random chance.
-
Document all data analysis steps and methodologies. This ensures transparency and reproducibility of the analysis.
Presenting Data Effectively
Clear and concise data presentation is vital for effective communication of poll results.
- Misleading visualizations: Poorly designed charts and graphs can distort the data and lead to misinterpretations.
- Lack of context: Presenting data without sufficient context can be misleading and incomplete.
Effective solutions include:
-
Interactive dashboards: Interactive dashboards allow users to explore the data in detail and customize their views.
-
Clear labeling and annotation of charts: All charts and graphs should be clearly labeled and annotated to provide context and avoid ambiguity.
-
Avoiding misleading visualizations: Avoid using techniques that distort the data or create a false impression.
-
Use infographics to communicate complex data simply. This improves understanding and engagement.
-
Choose appropriate chart types (bar charts, pie charts, line graphs). Different chart types are suitable for different data types.
-
Provide clear context and explanations for all visualizations. This ensures that the data is interpreted correctly.
Security and Privacy Concerns in Poll Data Systems
Protecting the security and privacy of poll data is paramount, especially considering the sensitive nature of the information often collected.
- Data breaches: Unauthorized access to poll data can lead to identity theft, reputational damage, and legal repercussions.
- Privacy violations: Failure to protect respondent privacy can violate data protection regulations and erode public trust.
Addressing these concerns requires:
-
Data encryption: Encrypting data at rest and in transit protects it from unauthorized access.
-
Access control mechanisms: Implementing access control measures ensures that only authorized personnel can access the data.
-
Anonymization techniques: Anonymizing data protects respondent identities while preserving the integrity of the data for analysis.
-
Implement robust security protocols to protect data from unauthorized access. This includes firewalls, intrusion detection systems, and regular security audits.
-
Obtain informed consent from respondents. This ensures that respondents are aware of how their data will be used and protected.
-
Comply with all relevant data privacy regulations. This includes regulations such as GDPR and CCPA.
Conclusion
Building a robust poll data system requires careful consideration of various challenges related to data acquisition, analysis, and security. By implementing the solutions outlined in this article – from employing rigorous sampling methodologies and utilizing advanced data management techniques to prioritizing data security and adhering to privacy regulations – organizations can significantly improve the accuracy, reliability, and efficiency of their polling efforts. Accurate poll data is essential for informed decision-making across numerous sectors, and investing in a robust system is an investment in better outcomes. Start improving your polling processes today by implementing these best practices and building a robust poll data system for more accurate and reliable results. For further resources on data management and analysis tools, explore [link to relevant resources].
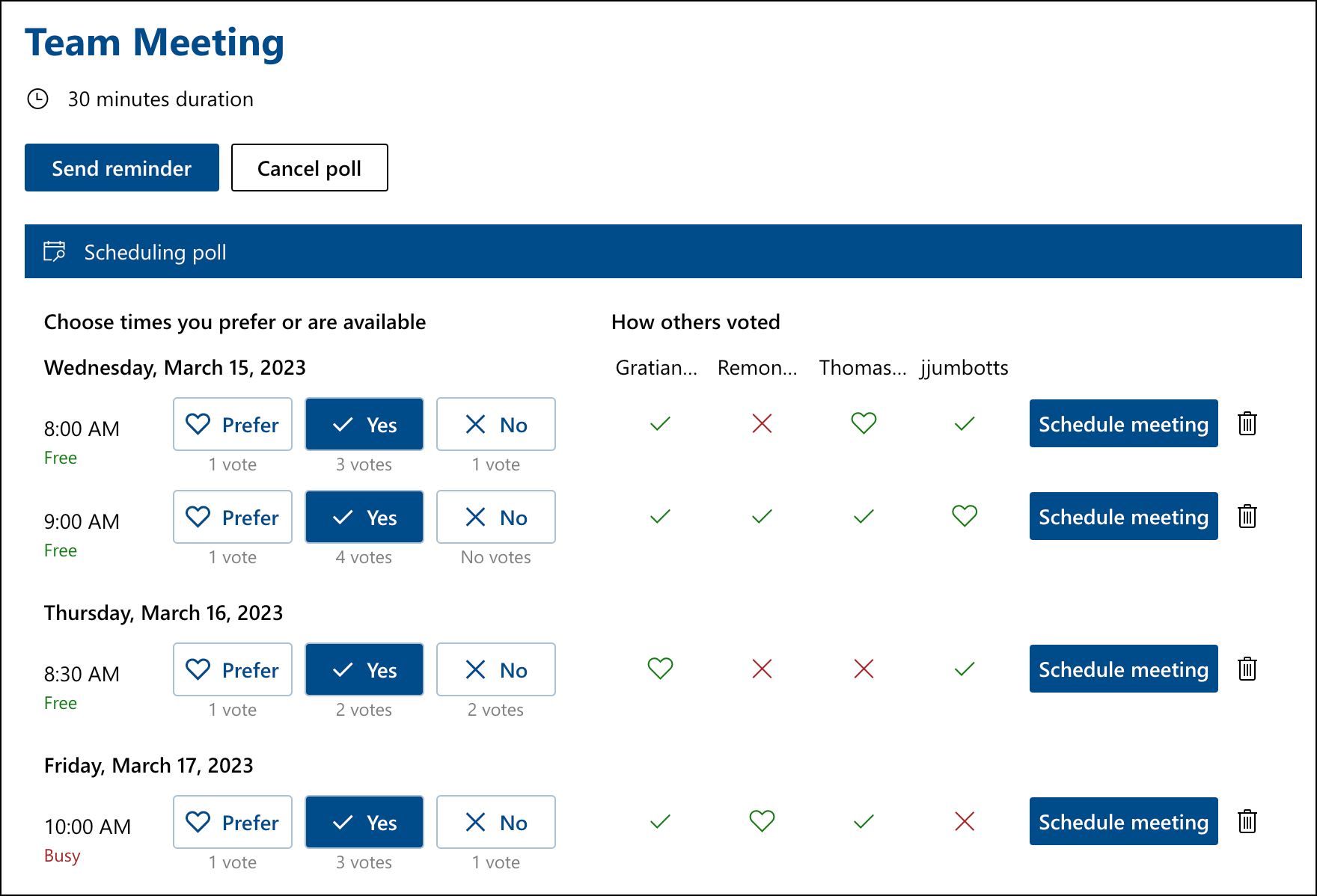
Featured Posts
-
Reform Uk Five Reasons Nigel Farages Party Faces Collapse
May 03, 2025 -
April 17 2025 Daily Lotto Results Announced
May 03, 2025 -
Are Fortnite Fans Displeased With The Latest Item Shop
May 03, 2025 -
The High Cost Of Neglect Investing In Childhood To Prevent Mental Health Issues
May 03, 2025 -
Lacrosse Hazing Investigation 11 Players Avoid Kidnapping Charges In Syracuse
May 03, 2025
Latest Posts
-
Watch The Chicago Cubs Vs La Dodgers Mlb Tokyo Series Live Online
May 04, 2025 -
Ufc 314 Main Event Odds A Deep Dive Into Volkanovski Vs Lopes
May 04, 2025 -
Ufc 314 Volkanovski Lopes Main Event Early Betting Odds
May 04, 2025 -
Direct To Consumer Streaming Fox Brings On Peter Distad
May 04, 2025 -
Volkanovski Vs Lopes Ufc 314 Main Event Opening Odds Breakdown
May 04, 2025