Debunking The Myth: How AI Processes Information Compared To Human Thought
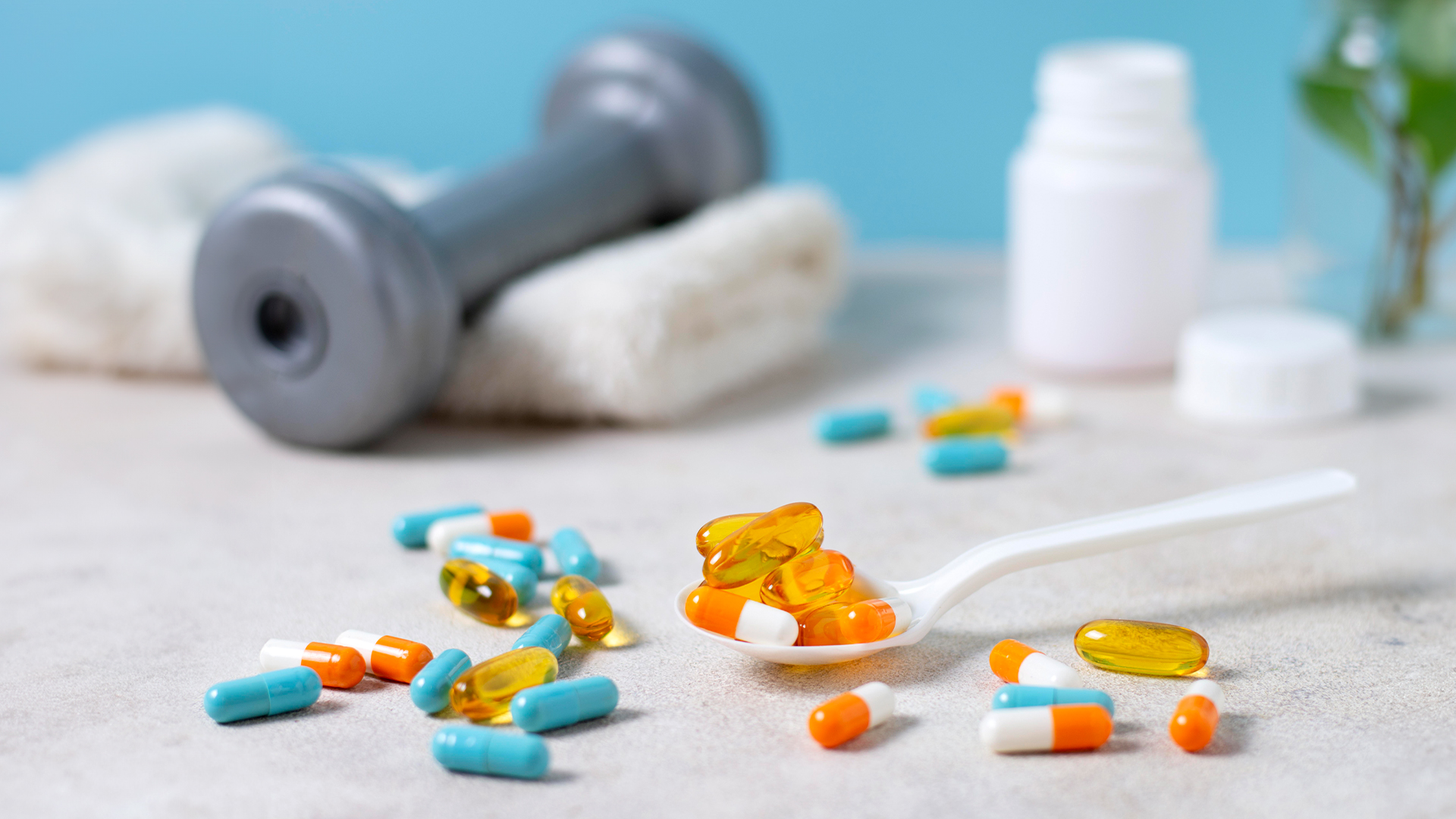
Table of Contents
The Algorithmic Nature of AI Information Processing
Data Dependence vs. Experiential Learning
A core difference lies in how AI and humans acquire knowledge. AI's information processing relies heavily on pre-existing data sets. Machine learning algorithms, the backbone of many AI systems, learn patterns and relationships within this data to make predictions or decisions. In contrast, humans learn through a rich tapestry of experiences, adaptation, and intuition.
- AI's dependence on training data: AI systems are only as good as the data they are trained on. Biased or incomplete data can lead to flawed outputs, highlighting a significant limitation in handling novel situations. An AI trained solely on images of cats, for instance, might fail to recognize a dog.
- Human capacity for abstract thought: Humans possess a remarkable ability for abstract thought, generalization, and creative problem-solving. We can learn from limited examples and apply knowledge to new contexts – a capacity largely absent in current AI systems.
- AI struggles with ambiguous or incomplete data: AI often struggles with ambiguity and incomplete information. Human cognition, however, can often fill in missing pieces or interpret vague information using prior knowledge and contextual cues.
- Human problem-solving beyond data analysis: Consider a detective solving a murder mystery – they use deduction, intuition, and understanding of human behavior, far exceeding simple data analysis. AI excels at analyzing data, but often lacks the broader contextual understanding to replicate such feats.
Parallel Processing vs. Serial Processing
Another key distinction lies in the way information is processed. AI excels at parallel processing, performing numerous calculations concurrently. This allows AI to efficiently handle massive datasets and complex computations. Human information processing, on the other hand, is largely serial, with information processed sequentially.
- AI's concurrent calculations: AI can analyze millions of data points simultaneously, leading to remarkably fast processing speeds for specific tasks.
- Human's sequential processing: Human brains process information in a more linear fashion, focusing on one piece of information at a time. This can be slower but allows for deeper contextual understanding.
- Speed and efficiency trade-offs: While AI surpasses humans in speed for certain tasks, human sequential processing allows for nuanced understanding and critical evaluation that AI often lacks.
- Limitations in nuanced understanding: AI's parallel processing, while efficient, can hinder its ability to grasp subtle nuances, context, and emotional undertones present in human communication.
The Role of Context and Understanding in Information Processing
Contextual Awareness
Humans effortlessly integrate context into their understanding of information. We interpret non-verbal cues, emotions, and intentions with ease. AI, however, often struggles with such nuanced understanding. Its interpretations are primarily driven by explicit programming and the data it's been trained on.
- Human interpretation of non-verbal cues: We understand sarcasm, body language, and subtle shifts in tone, adding layers of meaning beyond explicit words.
- AI's reliance on explicit programming: AI needs explicitly programmed rules or a vast amount of labelled data to interpret such cues. This reliance can lead to misinterpretations.
- Examples of AI misinterpretations: AI chatbots have famously provided inappropriate or nonsensical responses due to a lack of contextual awareness.
- Challenges of imbuing AI with common sense: One of the biggest challenges in AI research is imbuing AI with "common sense" reasoning – the ability to make inferences and draw conclusions based on general knowledge of the world.
Common Sense Reasoning and Intuition
Humans possess common sense reasoning and intuition—abilities that are largely absent in current AI systems. We make inferences and assumptions based on our life experiences, a capability that AI lacks.
- Human inference and assumptions: We can intuitively understand that a wet street likely indicates recent rain, without needing explicit data to confirm it.
- AI's lack of independent development: AI cannot independently develop common sense or intuitive understanding. It needs to be explicitly programmed with such rules, which are often difficult to define comprehensively.
- Examples of AI failure: AI might struggle with tasks that require understanding of basic physical laws or social norms, areas where humans naturally excel.
- Research into incorporating common sense: Researchers are actively exploring ways to incorporate common sense reasoning into AI systems, often involving knowledge graphs and symbolic AI approaches.
The Future of AI and Human Collaboration
Synergistic Potential
Despite its limitations, AI holds tremendous potential when combined with human creativity and critical thinking. The future lies in synergistic collaborations where AI and humans complement each other's strengths.
- AI assisting humans: AI can assist humans in data analysis, complex problem-solving, and repetitive tasks, freeing up human time for more creative and strategic endeavors.
- Humans guiding AI development: Humans play a crucial role in guiding AI development, ensuring ethical considerations are addressed and biases are minimized.
- Examples of successful collaborations: We already see successful examples in medical diagnosis, financial modeling, and scientific research, where AI assists human experts.
Addressing Limitations
Ongoing research focuses on improving AI's ability to process information more like humans, bridging the gap between machine intelligence and human cognition.
- Advances in NLP and machine learning: Progress in natural language processing (NLP) and machine learning is continuously improving AI's ability to understand and generate human-like text and respond to complex queries.
- Development of more robust AI: Researchers are developing more robust and adaptable AI systems capable of handling uncertainty and incomplete information more effectively.
- Focus on Explainable AI (XAI): Explainable AI (XAI) aims to make AI decision-making processes more transparent and understandable, increasing trust and accountability.
Conclusion
In summary, how AI processes information differs significantly from human thought. AI excels at parallel processing vast datasets and performing specific tasks with remarkable speed and efficiency. However, it currently lacks the contextual awareness, common sense reasoning, and intuitive understanding that characterize human cognition. The unique strengths of both AI and human intelligence are best leveraged through collaboration. Understanding how AI processes information is crucial for responsible development and effective collaboration. Continue learning about the nuances of artificial intelligence and human cognition to harness the full potential of both.
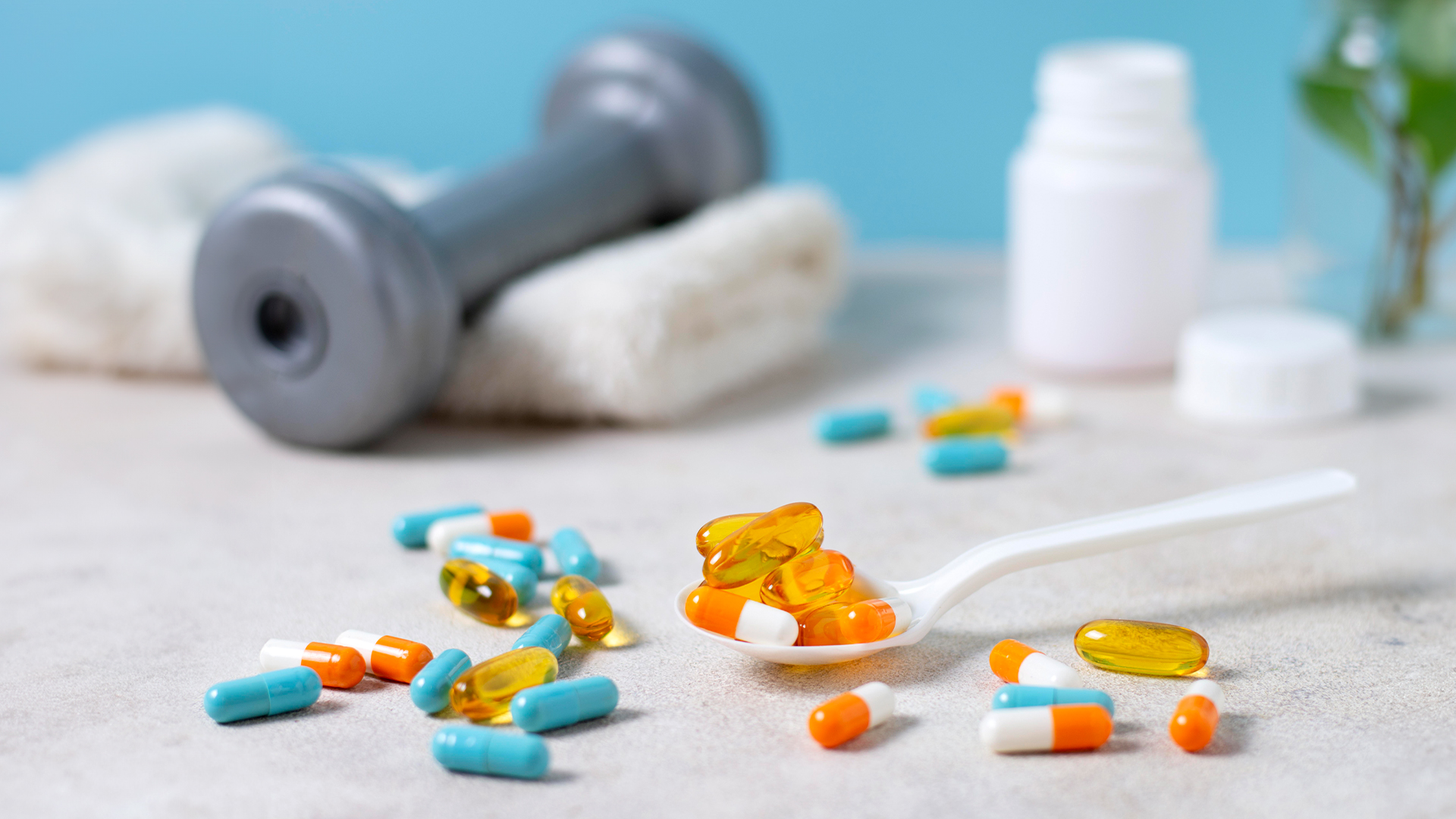
Featured Posts
-
Nyt Spelling Bee Solutions For March 15 2025 Find The Pangram
Apr 29, 2025 -
Albertas Economic Outlook Analyzing The Dow Project Delay
Apr 29, 2025 -
Dsv Leoben Neues Trainerteam Fuer Die Regionalliga Mitte
Apr 29, 2025 -
Transparency Concerns Raised Over Usps Mail Delays In Louisville
Apr 29, 2025 -
High Stock Market Valuations A Bof A Analysis And Investor Reassurance
Apr 29, 2025