How AI Works: A Look At The Mechanisms Behind AI "Thinking"
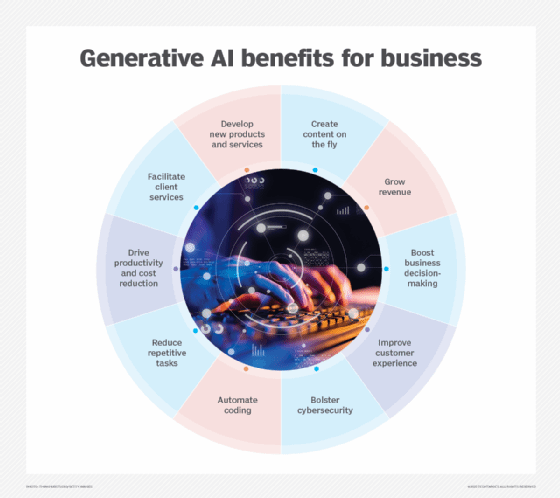
Table of Contents
The Foundation: Machine Learning (ML) and Deep Learning (DL)
Artificial Intelligence often relies on machine learning (ML) and its more advanced counterpart, deep learning (DL). While both involve teaching computers to learn from data without explicit programming, they differ significantly in their approach. Understanding how AI works starts with grasping these fundamental concepts.
Machine learning uses algorithms to parse data, learn from it, and make decisions or predictions based on what it has learned. Deep learning, a subset of machine learning, uses artificial neural networks with multiple layers to analyze data and extract complex features. Think of it like this: machine learning is a broad term, and deep learning is a powerful, specific type of machine learning.
Supervised Learning
Supervised learning is a type of machine learning where the algorithm learns from a labeled dataset. This means that each data point is tagged with the correct answer or outcome. For example:
- Image Classification: A model is trained on thousands of images labeled "cat" or "dog," learning to distinguish between them.
- Spam Filtering: Emails are labeled as "spam" or "not spam," teaching the algorithm to identify and filter spam messages.
The algorithm learns the relationship between the input data and the labels, enabling it to predict the correct label for new, unseen data. Common algorithms used in supervised learning include:
- Linear Regression
- Support Vector Machines (SVMs)
- Decision Trees
- Random Forests
Unsupervised Learning
Unsupervised learning deals with unlabeled data. The algorithm's task is to find patterns, structures, and relationships within the data without any pre-defined answers. Examples include:
- Clustering: Grouping similar data points together (e.g., customer segmentation based on purchasing behavior).
- Dimensionality Reduction: Reducing the number of variables while preserving important information (e.g., simplifying complex data for easier analysis).
Common algorithms in unsupervised learning are:
- K-means Clustering
- Principal Component Analysis (PCA)
- Hierarchical Clustering
Reinforcement Learning
Reinforcement learning is a type of machine learning where an agent learns to interact with an environment to maximize a reward. It's essentially trial-and-error learning. Examples are:
- Game Playing: An AI agent learns to play a game like chess or Go by playing repeatedly and receiving rewards for winning or penalties for losing.
- Robotics: A robot learns to navigate a complex environment by receiving rewards for completing tasks and penalties for errors.
Reinforcement learning algorithms often use:
- Q-learning
- SARSA (State-Action-Reward-State-Action)
Neural Networks: The Brain of AI
Artificial Neural Networks (ANNs) are at the heart of many AI systems. Inspired by the structure and function of the human brain, ANNs consist of interconnected nodes (neurons) organized in layers. Understanding how AI works requires a basic grasp of neural networks.
Structure and Function of ANNs
A typical ANN has three main layers:
- Input Layer: Receives the initial data.
- Hidden Layer(s): Process the data and extract features. There can be multiple hidden layers, increasing the network's complexity and learning capacity.
- Output Layer: Produces the final result (e.g., classification, prediction).
The process of feeding data through the network is called forward propagation, and adjusting the network's weights based on errors is called backpropagation. Activation functions introduce non-linearity, allowing the network to learn complex patterns.
Types of Neural Networks
Different types of neural networks are designed for specific tasks:
- Convolutional Neural Networks (CNNs): Excellent for image and video processing.
- Recurrent Neural Networks (RNNs): Ideal for processing sequential data like text and time series.
Data: The Fuel for AI
High-quality data is the lifeblood of AI. Without sufficient, accurate, and relevant data, even the most sophisticated algorithms will fail. How AI works is intrinsically linked to the quality and quantity of data it's trained on.
Data Collection and Preprocessing
Acquiring and cleaning data is a significant challenge. This involves:
- Gathering data from various sources.
- Handling missing values and outliers.
- Data augmentation (creating more data from existing data).
- Data normalization (scaling data to a consistent range).
Big Data and AI
The performance of AI algorithms significantly improves with larger datasets. Big data, characterized by its volume, velocity, and variety, fuels the advancement of AI. Cloud computing plays a crucial role in storing, processing, and analyzing the massive datasets required for training complex AI models.
AI Applications: Seeing AI in Action
AI is transforming numerous industries:
Specific examples:
- Healthcare: AI aids in disease diagnosis, drug discovery, and personalized medicine. Image analysis using CNNs helps radiologists detect tumors more accurately.
- Finance: AI powers fraud detection, algorithmic trading, and risk assessment. Machine learning algorithms analyze financial data to predict market trends.
- Transportation: Self-driving cars utilize AI for navigation, object recognition, and decision-making. Reinforcement learning helps these systems learn to navigate complex driving scenarios.
Understanding How AI Works – A Path to Innovation
This article has explored the key concepts behind how AI works, including machine learning, deep learning, neural networks, and the crucial role of data. While complex, the fascinating nature of AI lies in its ability to learn, adapt, and solve problems in ways previously unimaginable. Continue exploring the world of AI, learn more about how AI works, and discover the power of Artificial Intelligence to shape our future. Understand the intricacies of AI development and contribute to this rapidly evolving field.
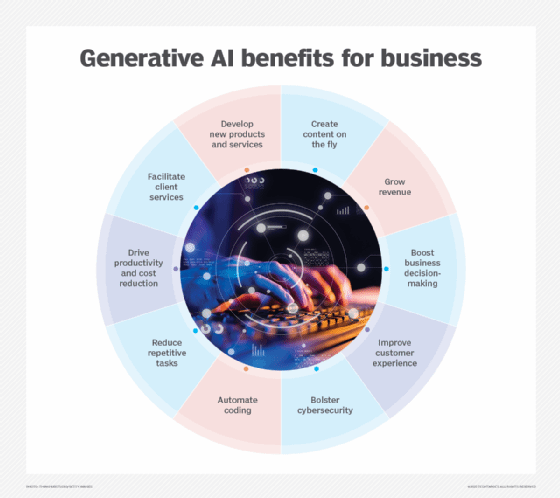
Featured Posts
-
Country Legend Willie Nelson Releases Oh What A Beautiful World
Apr 29, 2025 -
Car Ramming Attack On Canadian Filipino Community What We Know
Apr 29, 2025 -
Open Ai Facing Ftc Investigation Concerns Over Chat Gpts Data Practices
Apr 29, 2025 -
The Rise Of Wildfire Betting A Reflection Of Our Times
Apr 29, 2025 -
Us Researcher Exodus How Funding Cuts Fuel Global Talent Wars
Apr 29, 2025
Latest Posts
-
New Willie Nelson Album Celebrating 92 Years With Solo Release Number 77
Apr 29, 2025 -
Willie Nelsons 77th Studio Album Arrives Ahead Of His 92nd Birthday
Apr 29, 2025 -
New Documentary Showcases Willie Nelsons Respect For His Longtime Road Manager
Apr 29, 2025 -
Willie Nelson Honors His King Of The Road Crew In New Documentary
Apr 29, 2025 -
Willie Nelsons Oh What A Beautiful World A Deep Dive Into The New Album
Apr 29, 2025