How Outdated Business Apps Hamper AI Implementation
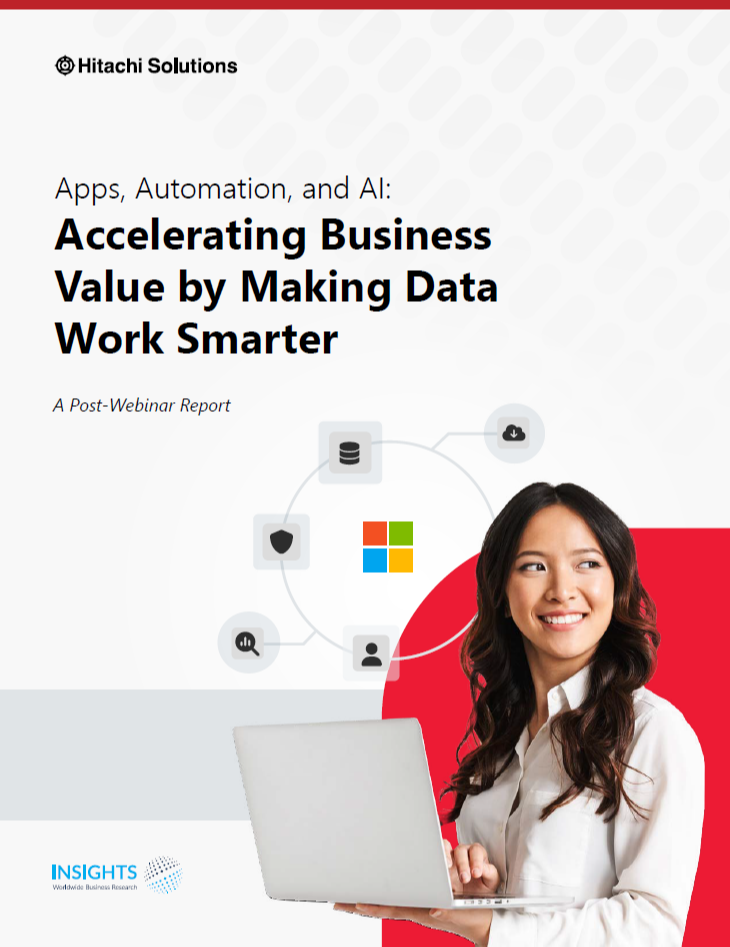
Table of Contents
Data Incompatibility and Integration Challenges
One of the most significant hurdles presented by outdated business apps is the challenge of data incompatibility and integration. Effective AI relies on large quantities of high-quality, readily accessible data. Unfortunately, legacy systems often create significant barriers to achieving this.
Data Silos and Lack of Interoperability
Outdated applications frequently result in data silos—isolated pockets of information trapped within disparate systems. This lack of interoperability makes it incredibly difficult to consolidate and analyze data for AI model training. The consequences are significant:
- Incompatible file formats: Legacy systems may use obsolete file formats that are not easily compatible with modern AI platforms and tools.
- Lack of APIs: Many outdated apps lack Application Programming Interfaces (APIs), making automated data extraction and integration incredibly challenging.
- Difficulties in data extraction and transformation: Manually extracting and transforming data from various legacy systems is time-consuming, expensive, and error-prone.
- Migration challenges: Moving data from legacy systems to cloud-based AI platforms often proves to be a complex and costly undertaking, requiring specialized expertise and significant time investment.
Data Quality Issues
Beyond the issue of data silos, outdated systems often lack robust data validation and cleansing mechanisms. This leads to poor data quality—inaccurate, incomplete, or inconsistent data—which severely impacts the accuracy and reliability of AI models.
- Inaccurate, incomplete, or inconsistent data: Legacy systems may contain errors, missing values, and inconsistencies that are difficult to identify and correct.
- Difficulties in error correction: Identifying and correcting errors in large, legacy databases can be a monumental task, requiring significant manual effort and expertise.
- Impact on AI model accuracy: Poor data quality directly translates to inaccurate AI model predictions, rendering the AI solution ineffective and potentially leading to costly business mistakes.
Limited Scalability and Performance Issues
Outdated business applications are often built on outdated infrastructure that simply cannot handle the computational demands of modern AI algorithms. This lack of scalability and performance significantly hinders AI implementation.
Infrastructure Constraints
Legacy systems frequently suffer from limitations in processing power, storage capacity, and scalability. This impacts AI implementation in several ways:
- Slow processing speeds: Outdated hardware struggles to process the large datasets required for effective AI model training, significantly increasing training times.
- Limited storage capacity: Legacy systems may lack the storage capacity to accommodate the growing volume of data generated by modern businesses.
- Lack of scalability: As data volumes increase, outdated systems struggle to scale, resulting in performance bottlenecks and hindering AI model development.
Security Risks and Compliance Issues
Another significant concern is the heightened security risks associated with outdated applications. Many legacy systems lack the robust security features necessary to protect sensitive data, particularly crucial when implementing AI solutions that often handle vast amounts of personal or confidential information.
- Vulnerabilities to cyberattacks: Outdated software is vulnerable to known security exploits, increasing the risk of data breaches and cyberattacks.
- Difficulties in regulatory compliance: Meeting modern data protection regulations, such as GDPR, is challenging with legacy systems that lack built-in compliance features.
- Reputational and financial damage: Data breaches and non-compliance can result in significant reputational damage, hefty fines, and legal repercussions.
Lack of Automation and Workflow Integration
Outdated applications often require significant manual intervention, creating bottlenecks and hindering the automation capabilities of AI.
Manual Processes and Bottlenecks
Legacy systems frequently necessitate manual data entry, processing, and analysis, which is time-consuming and inefficient. This severely limits AI's potential to automate tasks and improve overall productivity.
- Time-consuming manual processes: Manual data entry and processing create significant bottlenecks in workflows.
- Reduced productivity: Manual processes drastically reduce overall productivity and increase the likelihood of human error.
- Lost opportunities for automation: Outdated apps prevent the automation of tasks that could be easily handled by AI, leading to lost opportunities for increased efficiency and cost savings.
Difficulty in Integrating with AI Tools
Integrating outdated apps with modern AI tools and platforms presents significant challenges. This often results in a fragmented technology stack, hindering the effectiveness of the AI solution.
- API limitations: Limited or non-existent APIs make it difficult to connect outdated applications with AI services.
- Incompatibility with cloud-based AI services: Many legacy systems are not designed to work with modern cloud-based AI platforms.
- Difficult custom integrations: Developing custom integrations to connect outdated apps with AI tools can be expensive, time-consuming, and technically complex.
Conclusion
Outdated business apps present significant challenges to successful AI implementation. Data incompatibility, limited scalability, and a lack of automation severely hinder the ability of organizations to leverage the full power of AI. The consequences include missed opportunities for increased efficiency, reduced competitiveness, and increased security risks. To unlock the true potential of AI, businesses must urgently assess their existing technology infrastructure and identify outdated applications that are hindering their AI initiatives. Investing in software modernization, upgrading to modern applications, and selecting solutions that facilitate seamless AI integration are crucial steps towards achieving successful AI adoption and driving significant business growth. Don't let outdated business apps stifle your growth – embrace digital transformation and the power of AI!
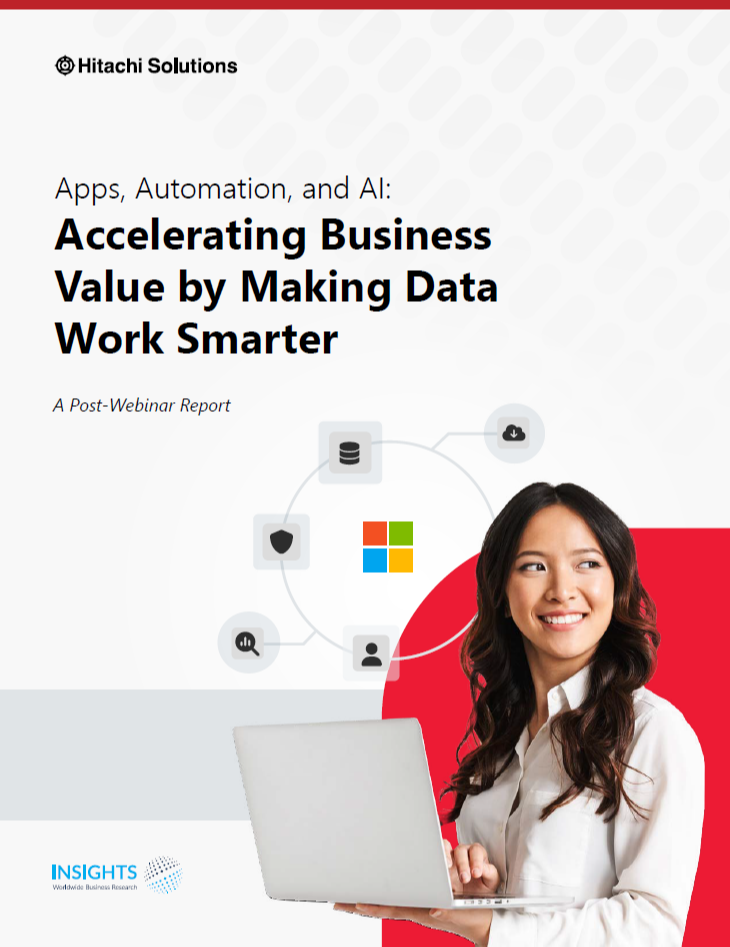
Featured Posts
-
Nieuw Schoolgebouw Kampen Stroomnet Aansluiting In Kort Geding
May 01, 2025 -
Impact Of River Road Construction On Louisvilles Restaurant Industry
May 01, 2025 -
Ripple Vs Sec 50 M Settlement Impact And Xrp Price Predictions
May 01, 2025 -
Hollywood Mourns Dallas And Carrie Actress Passes Away
May 01, 2025 -
The Merrie Monarch Festival Hoike A Deep Dive Into Pacific Island Arts
May 01, 2025
Latest Posts
-
Duponts Masterclass Frances Victory Over Italy
May 02, 2025 -
Englands Dramatic Late Victory Against France
May 02, 2025 -
Six Nations Frances Championship Win Thanks To Ramoss Leadership
May 02, 2025 -
France Six Nations Champions Scotland Defeat Highlights Ramoss Influence
May 02, 2025 -
Scotland Vs France Ramoss Key Role In Frances Six Nations Win
May 02, 2025