Improving The Accuracy Of Automated Visual Inspection Systems For Lyophilized Vials
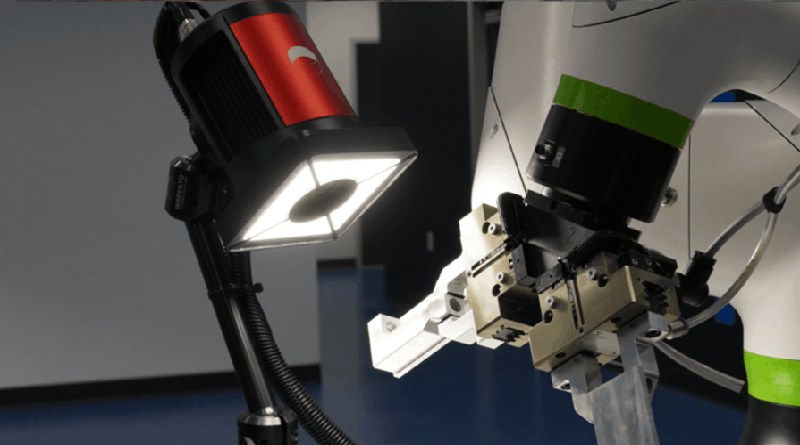
Table of Contents
Optimizing Image Acquisition for Enhanced Accuracy
High-quality images are the foundation of accurate automated visual inspection. Optimizing image acquisition involves careful consideration of lighting, camera selection, and image pre-processing techniques.
Lighting and Illumination Techniques
Appropriate lighting is paramount for effective defect detection. Different lighting techniques offer unique advantages and disadvantages:
- Coaxial lighting: Provides uniform illumination, minimizing shadows and highlighting surface imperfections like cracks or scratches. However, it may struggle to detect subsurface defects.
- Diffuse lighting: Offers softer illumination, reducing glare and highlighting subtle variations in color or texture. It can be less effective at detecting sharp, defined defects.
- Dark-field lighting: Excellent for detecting particulate matter and other small defects by illuminating scattered light. However, it may be less effective for larger defects or variations in surface texture.
The choice of lighting technique depends heavily on the types of defects expected and the characteristics of the lyophilized product. Uniform illumination is crucial to minimize shadows, which can obscure defects and lead to false negatives. Careful calibration of lighting intensity is also essential for consistent image quality.
Camera Selection and Resolution
Selecting the right camera is crucial for capturing the fine details necessary for accurate defect detection. High-resolution cameras with appropriate sensor size and sensitivity are essential.
- CCD vs. CMOS: CCD cameras generally offer higher image quality and lower noise, but CMOS cameras are often faster and more energy-efficient. The optimal choice depends on the specific application and priorities.
- Resolution and Defect Detection: Higher resolution directly translates to the ability to detect smaller defects. The resolution should be chosen to be sufficient to detect the smallest clinically relevant defects.
- Camera Positioning and Angle: Precise camera positioning and angle are vital for consistent image acquisition. Slight variations can significantly impact the visibility of defects.
Image Pre-processing Techniques
Image pre-processing steps enhance image quality and improve the accuracy of defect detection algorithms.
- Noise Reduction: Algorithms like median filtering or wavelet denoising remove unwanted noise, enhancing the clarity of the image.
- Image Enhancement: Techniques like contrast stretching or histogram equalization improve the visibility of defects by increasing contrast and sharpness.
- Artifact Mitigation: Careful selection and optimization of pre-processing parameters are critical to avoid introducing artifacts that might be misinterpreted as defects.
Advanced Algorithm Development for Defect Detection
The algorithms used for defect detection are crucial for the accuracy of AVIS. Modern approaches utilize machine learning and deep learning to improve performance.
Machine Learning and Deep Learning Approaches
Machine learning (ML) and deep learning (DL) offer significant advantages over traditional image processing methods for defect detection in lyophilized vials.
- Convolutional Neural Networks (CNNs): CNNs are particularly well-suited for image analysis, automatically learning complex features from large datasets.
- Training Data: The accuracy of ML/DL models is highly dependent on the quality and quantity of training data. A large, representative dataset is crucial for achieving high accuracy.
- Rare Defect Detection: A key challenge is detecting rare defects, requiring careful data augmentation and potentially specialized algorithms.
Feature Extraction and Classification
Effective feature extraction and classification are essential for accurate defect detection.
- Feature Types: Features extracted from images can include texture features (e.g., Gabor filters), shape features (e.g., circularity, aspect ratio), and color features (e.g., hue, saturation, value).
- Classification Algorithms: Algorithms like support vector machines (SVMs), random forests, and k-nearest neighbors (k-NN) can be used to classify features as defects or non-defects.
- Performance Evaluation: Metrics such as precision, recall, F1-score, and area under the ROC curve (AUC) are used to evaluate the performance of the classification models.
Handling Variations in Lyophilized Vials
Variations in fill level and cake appearance pose challenges for AVIS.
- Fill Level Compensation: Algorithms can be designed to compensate for variations in fill level by normalizing the image or using region-of-interest (ROI) analysis.
- Cake Appearance Compensation: Techniques such as image registration and texture analysis can be used to account for variations in cake appearance.
- Data Augmentation: Augmenting the training data with variations in fill level and cake appearance improves model robustness and generalizability.
Validation and Quality Control for Automated Systems
Rigorous validation and quality control are crucial for ensuring the accuracy and reliability of AVIS.
Establishing Robust Validation Protocols
Validation protocols must ensure the accuracy, precision, and repeatability of the AVIS.
- Accuracy: The ability of the system to correctly identify defects.
- Precision: The consistency of the system's measurements.
- Repeatability: The ability of the system to produce the same results under the same conditions.
- Regulatory Compliance: Validation must comply with relevant regulatory guidelines, such as those from the FDA.
Continuous Monitoring and Performance Evaluation
Continuous monitoring and evaluation are essential for maintaining system accuracy over time.
- Performance Metrics: Key metrics include the defect detection rate, false positive rate, and false negative rate.
- Performance Tracking: Regularly monitoring these metrics helps identify potential issues and maintain system performance.
- Maintenance and Calibration: Regular maintenance and calibration are critical to ensure the long-term accuracy of the AVIS.
Conclusion
Improving the accuracy of Automated Visual Inspection Systems for Lyophilized Vials is crucial for maintaining product quality and patient safety. By optimizing image acquisition techniques, developing advanced algorithms leveraging machine learning and deep learning, and implementing robust validation protocols, the pharmaceutical industry can significantly enhance the reliability and efficiency of AVIS. Continuous improvement and innovation in these areas are essential for the widespread adoption and success of Automated Visual Inspection Systems for Lyophilized Vials. Investing in advanced Automated Visual Inspection Systems for Lyophilized Vials will lead to significant improvements in efficiency, accuracy, and ultimately, patient safety.
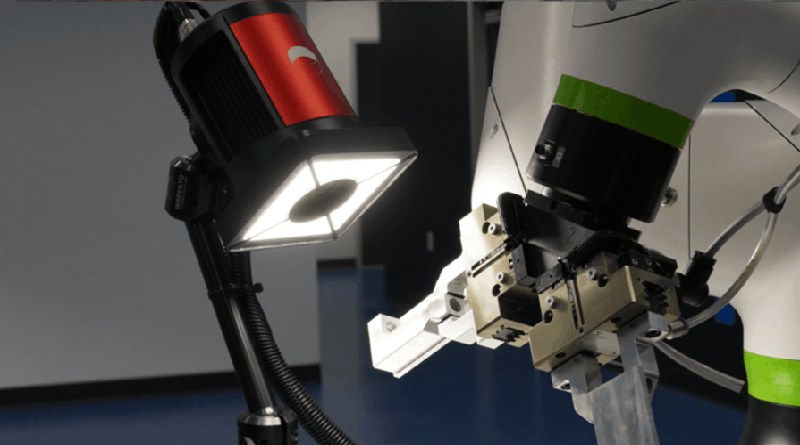
Featured Posts
-
Bundesliga Celebration Bayern Munichs Win And Muellers Home Goodbye
May 12, 2025 -
Undercover Sting Operation O Keefes Report Adds To Prince Andrews Legal Challenges
May 12, 2025 -
Grand Slam Track Kingston Where To Stream And Watch The Races
May 12, 2025 -
Knicks Edge Bulls In Double Overtime Thriller
May 12, 2025 -
Shane Lowrys Joy For Rory Mc Ilroy A Friends Unwavering Support
May 12, 2025