Legacy Systems And AI: A Recipe For Disaster?
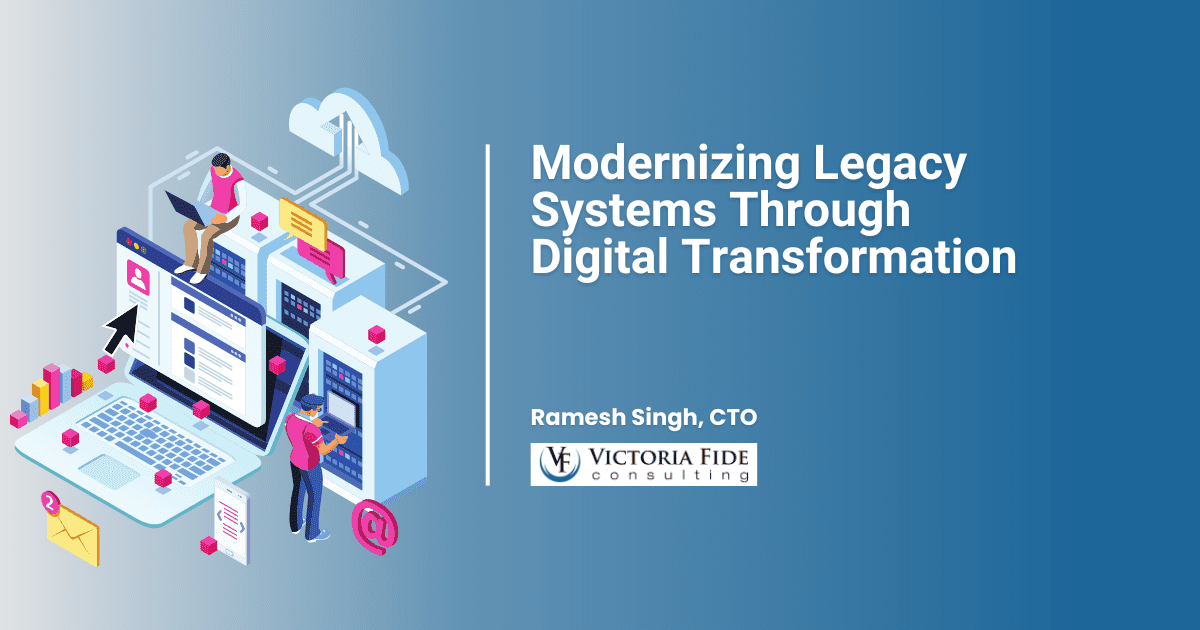
Table of Contents
Data Incompatibility: The Foundation of Failure
The foundation of many failed AI integration projects lies in data incompatibility. Legacy systems often house data in disparate, siloed formats, presenting significant hurdles for AI model training and implementation.
Data Silos and Data Migration Challenges
Extracting and integrating data from legacy systems is a major undertaking, fraught with challenges. These systems, often poorly documented and maintained, frequently contain data in inconsistent formats, lacking the metadata necessary for effective AI processing.
- Data format inconsistencies: Data might be stored in various formats (e.g., flat files, relational databases, proprietary formats), requiring extensive data transformation before it can be used by AI algorithms.
- Lack of metadata: Without proper metadata, understanding the context and meaning of the data becomes extremely difficult, hindering the accuracy and reliability of AI models.
- Security concerns during migration: Moving sensitive data from legacy systems to new AI environments requires robust security protocols to prevent breaches and maintain compliance.
- High cost and time investment: Data migration from legacy systems is a resource-intensive process, often requiring significant investment in time, personnel, and specialized tools. This adds significantly to the overall cost of legacy system data migration and data silo integration.
Data Quality Issues in Legacy Systems
In addition to format inconsistencies, legacy systems frequently contain inaccurate, incomplete, or outdated data. This poor data quality directly impacts the performance and accuracy of AI models.
- Data cleansing challenges: Cleaning and preparing legacy data for AI use often involves extensive manual intervention, further increasing costs and timelines.
- Impact of poor data quality on AI accuracy: Garbage in, garbage out – inaccurate data leads to inaccurate AI model predictions and undermines the value of the AI investment.
- Need for robust data validation processes: Implementing rigorous data validation processes is crucial to ensure the quality and reliability of the data used for AI model training. This necessitates careful consideration of data quality for optimal AI model training and AI data compatibility.
Integration Complexity: A Technological Minefield
Integrating AI with legacy systems presents significant technological challenges due to architectural mismatches and inherent system limitations.
Architectural Mismatches and Integration Hurdles
Modern AI architectures are typically agile and scalable, while legacy systems are often rigid and monolithic. Bridging this gap requires significant effort and expertise.
- API limitations: Legacy systems may lack the Application Programming Interfaces (APIs) needed for seamless integration with modern AI platforms.
- Scalability issues: Legacy systems may struggle to handle the increased data volume and processing demands associated with AI applications.
- Lack of system documentation: Poor or nonexistent documentation further complicates the integration process, increasing the risk of errors and delays.
- Potential for system conflicts: Integrating new AI technologies with legacy systems can lead to unforeseen conflicts and system failures if not carefully managed. Addressing legacy system integration challenges requires careful planning and execution.
Security Risks and Vulnerabilities
Integrating AI into legacy systems introduces new security risks, particularly if the legacy systems have outdated security protocols.
- Data breaches: Legacy systems may lack the security features necessary to protect sensitive data used in AI applications, increasing the risk of breaches.
- Vulnerabilities to cyberattacks: Outdated systems are more vulnerable to various cyberattacks, potentially compromising the entire AI infrastructure.
- Compliance issues: Integrating AI with legacy systems may create compliance issues, particularly regarding data privacy and security regulations.
- Increased attack surface: The integration process itself increases the overall attack surface, making the system more vulnerable to malicious actors. Careful consideration of legacy system security and AI security risks is paramount.
Cost and Return on Investment (ROI): Weighing the Risks
The financial implications of integrating AI with legacy systems are significant, requiring careful consideration of both upfront costs and the potential return on investment.
High Upfront Costs of Modernization
Modernizing legacy systems to support AI integration demands substantial financial investment.
- Cost of data migration: Migrating data from legacy systems is expensive, involving costs associated with data cleansing, transformation, and migration tools.
- System upgrades: Upgrading legacy systems to meet the requirements of AI integration can involve significant hardware and software costs.
- Software development: Developing custom software solutions to bridge the gap between legacy systems and AI platforms can be costly and time-consuming.
- Consulting fees: Hiring expert consultants to guide the integration process can add substantial costs to the project. Understanding the full impact of legacy system modernization and AI integration costs is crucial.
Uncertain Return on Investment
Despite the high upfront costs, the return on investment for AI projects involving legacy systems is often uncertain.
- Project delays: Integration projects involving legacy systems can be subject to significant delays due to unforeseen technical challenges.
- Unexpected costs: Unforeseen issues can lead to significant cost overruns, exceeding the initial budget projections.
- Potential for low or negative ROI: If integration is poorly managed, the project might fail to deliver the expected benefits, resulting in a low or even negative ROI.
- Difficulty in measuring the impact of AI: Measuring the actual impact of AI on business outcomes can be challenging, making it difficult to assess the true ROI. Careful cost-benefit analysis is vital when considering AI ROI and legacy system upgrade ROI.
Conclusion
Integrating AI with legacy systems presents a complex challenge with significant potential for cost overruns, delays, and project failure. Data incompatibility, integration complexity, and uncertain ROI are major hurdles that must be carefully addressed. Thorough planning, robust data quality assessment, and a phased, iterative approach are crucial for success. Don't let legacy systems and AI become a recipe for disaster! Carefully evaluate your options, prioritize data quality, and plan strategically for a successful integration. Seek expert advice to navigate these complexities and ensure a smooth transition to a future where legacy systems and AI work together effectively.
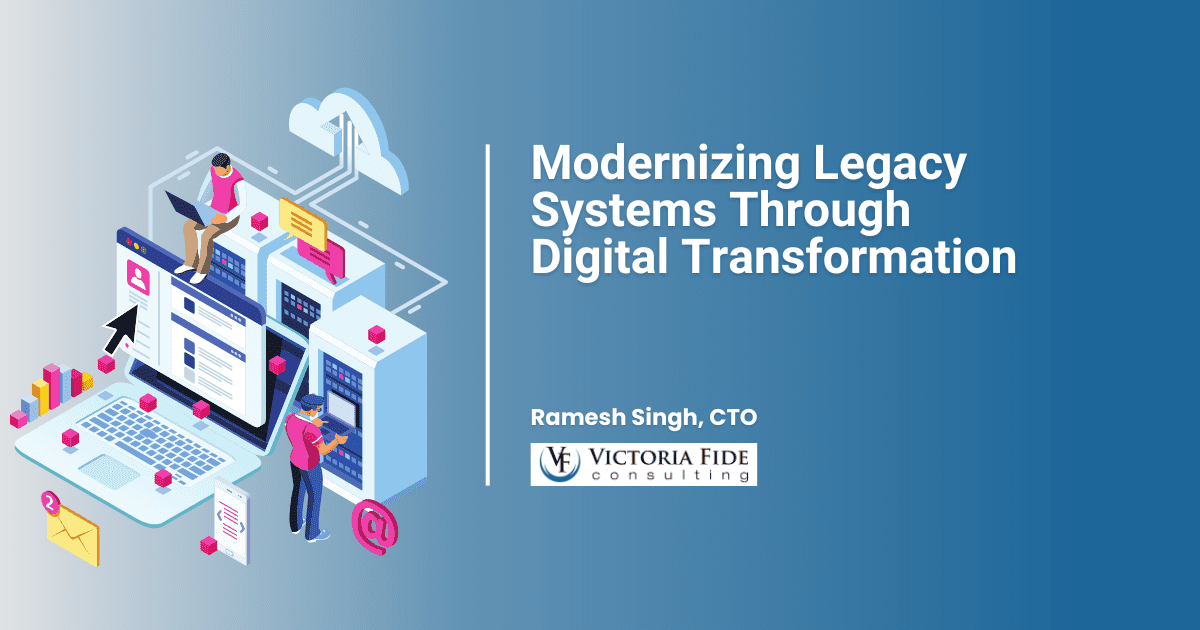
Featured Posts
-
Episima I Pari Stin Euroleague Kai Tin Epomeni Sezon
May 01, 2025 -
Death Of Dallas Legend At 100
May 01, 2025 -
Little Coffee A Dragons Den Success Story Four Investment Offers
May 01, 2025 -
Omni Wins Dragons Den Investment For Plant Based Dog Food
May 01, 2025 -
Michael Sheens 1 Million Debt Relief For 900 People
May 01, 2025
Latest Posts
-
Rugby World Cup Duponts Dominant Performance Against Italy
May 02, 2025 -
Duponts Masterclass Frances Victory Over Italy
May 02, 2025 -
Englands Dramatic Late Victory Against France
May 02, 2025 -
Six Nations Frances Championship Win Thanks To Ramoss Leadership
May 02, 2025 -
France Six Nations Champions Scotland Defeat Highlights Ramoss Influence
May 02, 2025