Turning "Poop" Into Podcast Gold: An AI-Powered Approach To Repetitive Documents
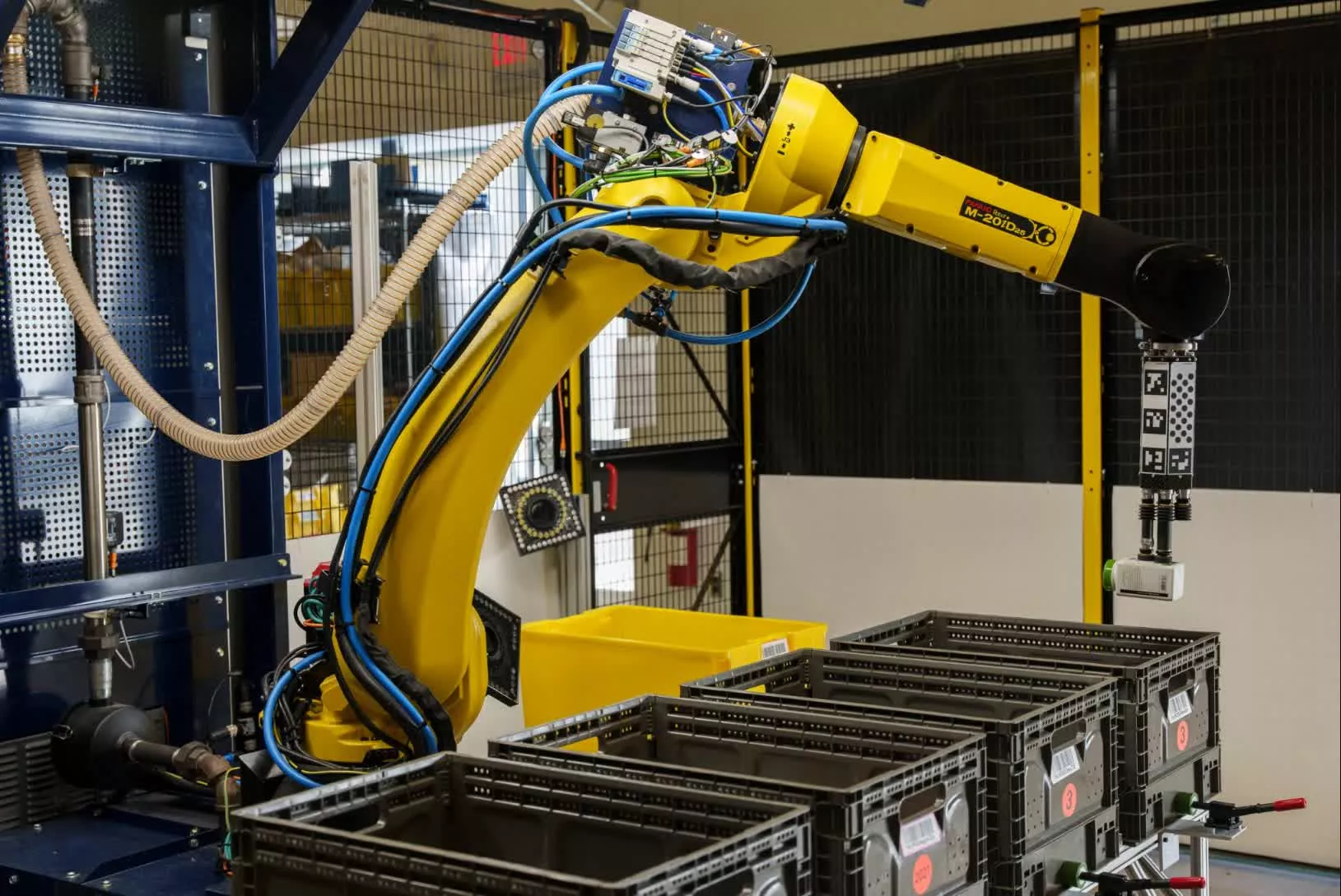
Table of Contents
Identifying and Quantifying Data Redundancy ("Poop"):
Defining the Problem:
What constitutes repetitive documents? Data redundancy, in its many forms, represents a significant hurdle for businesses of all sizes. It manifests in various ways, creating inefficiencies and hindering effective decision-making. Examples include:
-
Duplicate client records: Multiple entries for the same client scattered across different databases or spreadsheets.
-
Similar meeting minutes: Slightly altered versions of the same meeting notes stored in different locations.
-
Redundant data entries in spreadsheets: Repeated information within a single spreadsheet or across multiple spreadsheets.
-
Identical images or files: Multiple copies of the same image or document taking up unnecessary storage space.
-
Manual identification is time-consuming and prone to error: Manually identifying and removing duplicate data is incredibly labor-intensive and often results in human error, leading to incomplete or inaccurate data cleaning.
-
The cost of data redundancy: This extends beyond simply wasted storage space. It includes the cost of processing and analyzing redundant data, leading to inefficiencies and increased IT expenditure.
-
AI's role in quickly and accurately pinpointing redundant information: Artificial intelligence (AI) offers a powerful solution, capable of swiftly and accurately identifying and quantifying data redundancy, significantly reducing the time and resources needed for data cleaning and deduplication.
AI-Powered Solutions for Data Deduplication:
Natural Language Processing (NLP):
How NLP algorithms identify semantic similarities in text-based documents, even if the wording differs slightly. NLP is a crucial component in tackling text-based data redundancy. It goes beyond simple keyword matching, focusing on the meaning and context of the text.
- NLP techniques like stemming, lemmatization, and cosine similarity: These techniques allow NLP algorithms to understand the relationships between words and phrases, identifying duplicates even if they are phrased differently. Stemming reduces words to their root form (e.g., "running" to "run"), while lemmatization considers the context to identify the dictionary form (e.g., "better" to "good"). Cosine similarity measures the angular distance between document vectors, indicating semantic similarity.
- Examples of AI tools leveraging NLP for document comparison and deduplication: Several software solutions now integrate advanced NLP algorithms, enabling automated comparison and deduplication of text documents.
- Benefits: Improved accuracy, reduced manual effort, and faster processing times: By automating the process, AI-powered NLP solutions deliver far greater accuracy than manual methods, significantly reducing manual effort and speeding up the entire deduplication process.
Machine Learning (ML) for Pattern Recognition:
How ML models learn to identify recurring patterns and anomalies within datasets to flag redundant data. Machine learning algorithms excel at identifying patterns and anomalies within datasets. They can be trained to recognize specific types of data redundancy, allowing for highly effective deduplication.
- Supervised and unsupervised learning techniques for identifying duplicates: Supervised learning uses labeled data to train the model, while unsupervised learning allows the model to identify patterns on its own. Both approaches are valuable in identifying duplicates.
- Training ML models on specific document types for better accuracy: Training ML models on specific document types (e.g., invoices, customer records) improves accuracy and efficiency.
- Applications: Identifying duplicate customer entries, eliminating redundant invoices, streamlining data entry: ML models can be deployed in various business contexts to automate data deduplication, improving data quality and efficiency.
Transforming "Poop" into Podcast Gold: Practical Applications & Benefits:
Streamlined Workflows:
How reduced data redundancy leads to improved efficiency and productivity. Eliminating data redundancy directly translates to a more streamlined workflow across your organization.
- Faster data analysis and reporting: With less data to process, analysis and reporting become significantly faster and more efficient.
- Reduced storage costs: Removing duplicate data frees up valuable storage space, reducing IT infrastructure costs.
- Improved data quality and accuracy: Deduplication ensures that you're working with a clean and accurate dataset, improving the reliability of your insights.
Enhanced Decision-Making:
How cleaner, deduplicated data supports better-informed business decisions. The benefits of data deduplication extend far beyond simple efficiency gains. Cleaner data leads to better decisions.
- More reliable market analysis: Accurate, deduplicated data provides a more reliable basis for market research and analysis.
- Improved customer segmentation: Clean customer data allows for more effective customer segmentation and targeted marketing campaigns.
- Better resource allocation: Reliable data supports better resource allocation and strategic planning.
Conclusion:
Turning mountains of repetitive documents ("poop") into valuable insights is achievable through AI-powered solutions. By leveraging NLP and ML, businesses can efficiently identify, eliminate, and transform redundant data into a goldmine of information, leading to significant improvements in efficiency, decision-making, and ultimately, the bottom line. Don't let data redundancy slow you down; embrace AI-driven data deduplication and turn your "poop" into podcast gold – or better yet, profitable, actionable insights. Start exploring AI-powered solutions for data deduplication today!
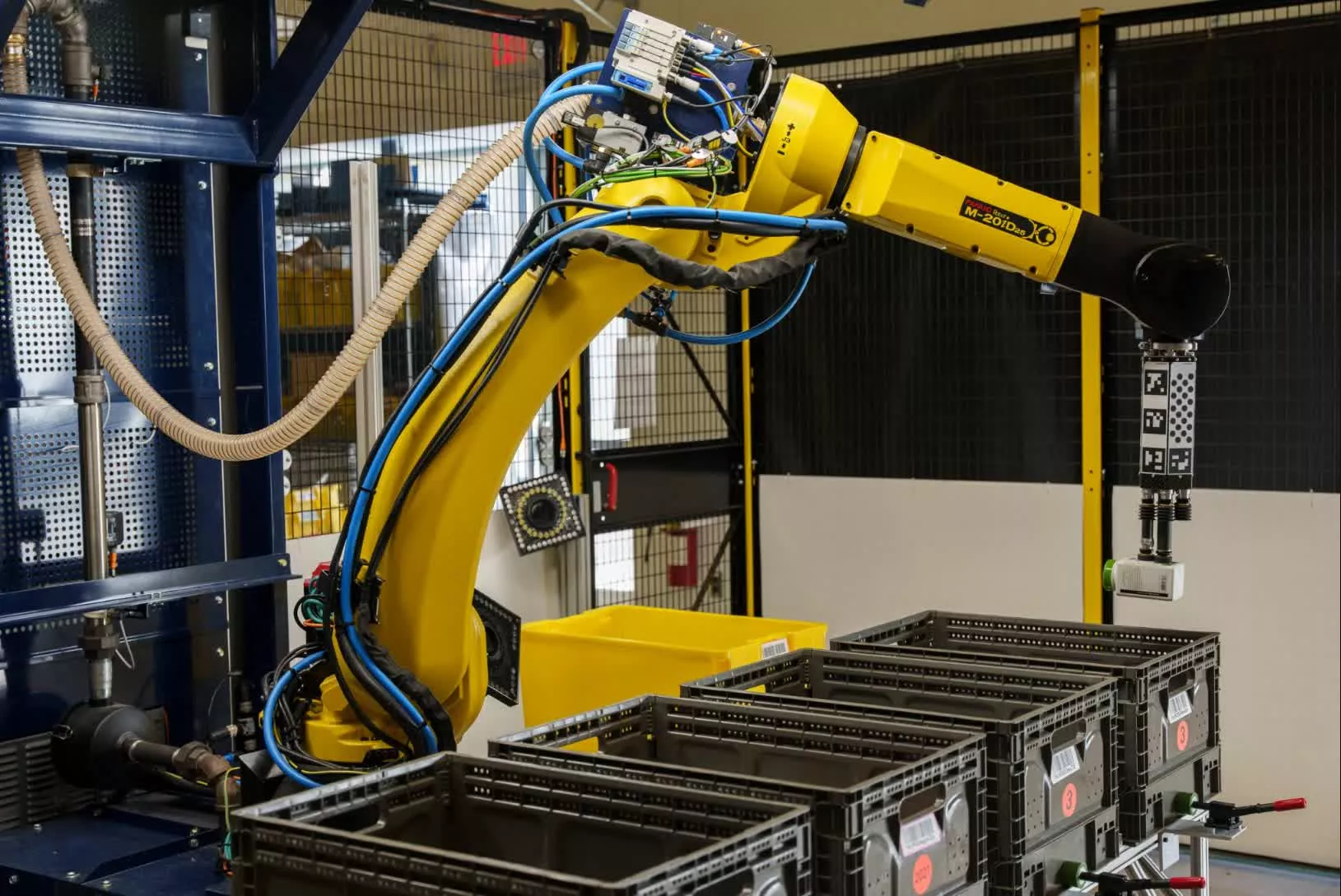
Featured Posts
-
Solidarnosc I Republika Porownanie Retoryczne Wg Sakiewicza Oraz Wyjatkowe Wyroznienia
May 02, 2025 -
Frances Six Nations Triumph Analyzing Mauvakas Performance And The Emerging Lions Squad
May 02, 2025 -
Analyzing Ripple Xrp Millionaire Potential In 2024
May 02, 2025 -
The Donkey Roundup A Southern California Community Event
May 02, 2025 -
Channel 4s Million Pound Giveaway Christopher Stevens Critique
May 02, 2025
Latest Posts
-
Newsround On Bbc Two Hd Your Complete Guide To Airtimes
May 02, 2025 -
Bbc Two Hd Newsround Tv Listings And Schedule
May 02, 2025 -
Live Tv Chaos Presenter Pulls Out Host Takes Over
May 02, 2025 -
A Travelers Guide To This Country
May 02, 2025 -
This Country Beyond The Tourist Trail
May 02, 2025