We Now Know How AI "Thinks"—and It's Barely Thinking At All
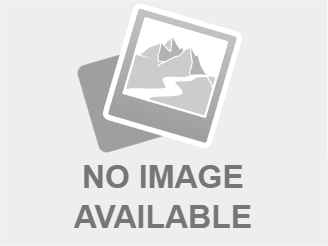
Table of Contents
The Illusion of Intelligence
The perception of AI intelligence often stems from its impressive ability to process and analyze vast amounts of data. However, a closer look reveals that AI's "thinking" is primarily based on sophisticated statistical pattern recognition, a far cry from human-like comprehension.
Statistical Pattern Recognition
AI, particularly in its most advanced forms, leverages powerful algorithms like deep learning and neural networks. These systems excel at identifying patterns within massive datasets. For example, image recognition systems learn to distinguish cats from dogs by identifying recurring patterns in millions of images. Similarly, natural language processing models learn to translate languages by recognizing statistical relationships between words and phrases.
- Deep Learning: Uses artificial neural networks with multiple layers to extract increasingly complex features from data.
- Convolutional Neural Networks (CNNs): Specialized for image and video processing, identifying patterns in visual data.
- Recurrent Neural Networks (RNNs): Designed for sequential data like text and speech, capturing temporal dependencies.
- Statistical Modeling: Underpins many AI algorithms, using probability and statistics to make predictions and classifications.
These techniques, while incredibly powerful, are fundamentally based on statistical probabilities and lack the semantic understanding inherent in human thought processes. The AI doesn't "understand" a cat; it identifies patterns consistent with what humans have labeled as "cat." This distinction is crucial to understanding the limitations of current AI thinking.
Lack of True Understanding
While AI can convincingly mimic human-like responses, it lacks genuine comprehension. It can manipulate language and data without understanding the underlying meaning. This limitation is often highlighted by AI generating nonsensical or illogical responses when presented with unexpected inputs or ambiguous queries.
- Example 1: An AI chatbot might correctly answer a question about the weather but fail to understand a nuanced philosophical question.
- Example 2: An AI image captioning system might accurately describe the visual elements of an image but misinterpret the overall context or meaning.
- Example 3: AI translation systems sometimes produce grammatically correct but semantically incorrect translations, demonstrating a lack of genuine understanding of the source language.
This lack of genuine understanding is a critical limitation of current AI's thought processes, highlighting the significant difference between sophisticated pattern recognition and true comprehension.
The Role of Data in AI "Thinking"
The "thinking" of an AI system is heavily reliant on the data it is trained on. This dependency creates both opportunities and significant challenges.
Data Bias and its Impact
AI systems are trained on massive datasets, and if these datasets contain biases, the AI will inevitably inherit and amplify those biases. This can lead to discriminatory or unfair outcomes in various applications.
- Facial Recognition Bias: Studies have shown that facial recognition systems perform less accurately on individuals with darker skin tones, reflecting biases in the training data.
- Loan Application Bias: AI-powered loan applications might unfairly discriminate against certain demographics if the training data reflects historical biases in lending practices.
- Algorithmic Bias: This broader term encompasses any systematic and repeatable errors in a computer system that create unfair outcomes, such as those stemming from biased training data.
Addressing data bias is crucial for ensuring fairness and ethical considerations in AI development and deployment. Mitigating AI bias requires careful curation of training datasets and the development of algorithms less susceptible to biased data.
The Limits of Extrapolation
AI systems are generally good at performing tasks within the scope of their training data. However, they often struggle when confronted with situations outside this scope. This limitation, often referred to as the problem of extrapolation, can lead to unexpected and incorrect behavior.
- Example 1: A self-driving car trained primarily on highway driving might perform poorly in complex urban environments.
- Example 2: A medical diagnosis AI trained on a specific population might be inaccurate when applied to a different demographic group.
- Out-of-distribution data: This refers to data that is significantly different from the data used to train the AI model, leading to poor performance.
Improving the ability of AI systems to generalize and extrapolate from their training data is a key area of ongoing research in artificial intelligence.
The Future of AI and "Thinking"
While current AI excels at pattern recognition, the future of AI "thinking" lies in developing systems with more genuine understanding and reasoning capabilities.
The Importance of Explainable AI (XAI)
To build trust and address concerns about bias and lack of comprehension, there is a growing need for explainable AI (XAI). XAI aims to create AI systems whose decision-making processes are transparent and understandable.
- Model Interpretability: Developing techniques to understand how a model arrives at its conclusions.
- Feature Importance Analysis: Identifying the key factors influencing a model's predictions.
- Rule Extraction: Extracting easily understandable rules from complex models.
XAI is critical for ensuring AI accountability and responsible innovation, allowing for better scrutiny of AI decision-making processes.
Beyond Statistical Pattern Recognition
Future research aims to move beyond purely statistical pattern recognition towards AI systems capable of genuine reasoning and understanding. This involves exploring several promising avenues:
- Symbolic AI: Using formal logic and symbols to represent knowledge and reason.
- Cognitive Architectures: Building AI systems inspired by human cognitive processes.
- Neuro-symbolic AI: Combining the strengths of neural networks and symbolic AI.
These research areas aim to develop AI systems with more robust capabilities, overcoming some of the current limitations of AI thinking.
Conclusion
In conclusion, the current state of AI "thinking" is primarily characterized by sophisticated statistical pattern recognition. While this enables impressive feats, it lacks the genuine understanding and reasoning capabilities of human intelligence. Current AI systems are also prone to biases reflecting the data they are trained on and struggle with situations outside the scope of their training. Understanding the limitations of how AI thinks is crucial for responsible innovation. Learn more about AI's capabilities and limitations, and join the conversation about building a more ethical and transparent future for artificial intelligence.
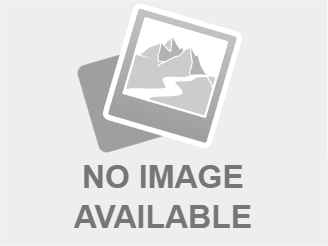
Featured Posts
-
Hagia Sophia A 1600 Year History Of Survival
Apr 29, 2025 -
Obstacles To Producing All American Products
Apr 29, 2025 -
Trump To Sign Executive Order Nationwide List Of Sanctuary Cities
Apr 29, 2025 -
Arizona Boating Competition Speedboats Daring Aerial Stunt
Apr 29, 2025 -
The Growing Appeal Of The Venture Capital Secondary Market
Apr 29, 2025
Latest Posts
-
New Willie Nelson Album Celebrating 92 Years With Solo Release Number 77
Apr 29, 2025 -
Willie Nelsons 77th Studio Album Arrives Ahead Of His 92nd Birthday
Apr 29, 2025 -
New Documentary Showcases Willie Nelsons Respect For His Longtime Road Manager
Apr 29, 2025 -
Willie Nelson Honors His King Of The Road Crew In New Documentary
Apr 29, 2025 -
Willie Nelsons Oh What A Beautiful World A Deep Dive Into The New Album
Apr 29, 2025